AILabs builds intelligence behind Taipei Traffic Density Network
Taipei is a city with millions of cars and motorcycles. Heavy traffic congestion occurs on the streets daily. Incidents have serious impact to traffic. Taipei have built tens of thousands citywide cameras recording real-time traffic videos. Police officers will consume the result to remove the traffic congestion. However, existing method requires human effort, only 16% of the incidents are manually detected.
Unlike cities in Mainland China, 2 major constraints exist on building the smart city systems in Taiwan. Humanity with Privacy and Integrity is the top priority. Taiwan is highly sensitive on human rights. The policy making need to ensure the goodwill with integrity. The protection of privacy and prevention of the future abuse and misuse together are the top priority on building the system. Taipei used videos with lower resolution that can’t further recognize residents’ identity in the video. Second, the solution need to be green and environmentally friendly. Forcing the city government to retire the old cameras is challenging. At the same time, Taipei city used low-frame-rate cameras to reduce the energy consumption. With these 2 constraints, Taiwan AILabs collaborated with Taipei City Government to build the autonomous traffic detection and prediction system.

Existing Traffic Cameras (CCTV) of Taipei City
The videos taken by the existing traffic cameras are low-resolution and low-frame-rate. We design a method with robust network to detect and predict the traffic congestion in real-time. We presented Taipei Traffic Density Network (TTDN). The network is able to precisely detect vehicle density of defined region in real-time. TTDN is a fully convolutional networks. In TTDN, we acquire multi-scale features from the images of traffic videos and obtain the estimation of vehicle density after pixel-wise regressions.
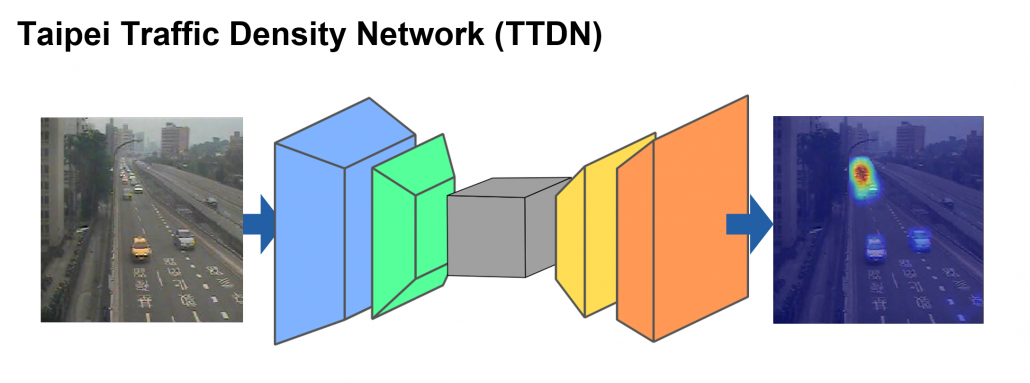
The Outline of Taipei Traffic Density Network (TTDN)
We would like to remark that our approach is intertwined with our key ingredients of smart city, protection of privacy and conservation of energy. Since the traffic videos are low-resolution, it’s hard to perform any face recognition or plate recognition. This ensure the protection of privacy. Meanwhile, on top of reusing existing traffic cameras, the data with low-resolution and low-frame-rate also reduce storage space and the computational cost.
Using the mathematical assumption of vehicle density map, we can efficiently detect and predict traffic congestion. Besides using density map directly, some existing works show that the density map is also a strong feature map. The fusion of density map and some other features may be able to detect accident, road construction, etc. This is an interesting topic we are currently working on.
The 2018 Smart City Summit & Expo (SCSE)